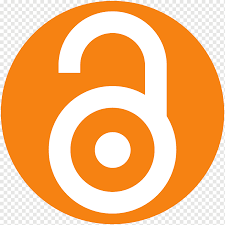
LIGHTWEIGHT RESIDUAL LAYERS BASED CONVOLUTIONAL NEURAL NETWORKS FOR TRAFFIC SIGN RECOGNITION
Babakulov Bekzod Mamatkulovich , Jizzakh Branch Of The National University, Teacher, UzbekistanAbstract
System for Traffic Sign Recognition and Classification is significantly important for especially traffic safety, traffic surveillance, artificial driver services and by all means, for self-driving cars. Traffic sign recognition plays an important role to tackle the traffic related obstacles. And, as traffic sign recognition is particularly applied to portable devices, lightweight models are essential aspect of the agenda. To overcome the mentioned problems, we propose lightweight convolutional neural networks with residual blocks based deep learning model for traffic recognition systems. We not only present the model efficiency but also show the several conducted experiments will well known deep CNN architectures over publicly available German traffic sign recognition benchmark. Our model showed 99.9 % accuracy by F-score, exceeding other models. At last, our model shows generally validity for traffic sign classification problem.
Keywords
Traffic surveillance, artificial driver services and by all means, for self-driving cars
References
S.K. Berkaya, H. Gunduz, O. Ozsen, C. Akinlar and S. Gunal, 2016. On circular traffic sign detection and recognition. Expert Systems with Applications, 48, pp.67-75.
Y. Yang, H. Luo, H. Xu and F. Wu. Toward real-time traffic sign detection and classification. IEEE Transactions on Intelligent transportation systems, 17(7), pp.2022-2031, 2015.
Y. LeCun, Y. Bengio. Convolutional networks for images, speech and time series – The handbook of brain theory and neural networks, 1995.
G. Loy, N. Barnes. Fast shape-based road sign detection for a driver assistance system. Intelligent Robots and Sytems, 2004. Proceedings, IEEE/RSJ International Conference on, 1, 70-75 vol.1.
W. Shaded, D. Abu-Al-Nadi, M. Mismar. Road traffic sign detection in color images. 10th IEEE International Conference on Electronics, Circuits and Systems, 2003.
Yang, WJ., Luo, CC., Chung, PC., Yang, JF.: Simplified Neural Networks with Smart Detection for Road Traffic Sign Recognition. Lecture Notes in Networks and Systems, vol 69. Springer, Cham (2020)
N. Barnes, G. Loy, D. Shaw. The regular polygon detector, 2010. Patter Recognition, 43, 592-602.
D.L. Escalera, L. Moreno, M. Salichs, J. Armiongol (1997). Road traffic sign detection and classification. IEEE Transactions on Industrial Electronics, 44, 848-859.
J. Stallkamp, M. Schlipsing, J. Salmen, C. Igel. The German traffic sign recognition benchmark: a multi-class classification competition. In the 2011 International joint conference on neural networks (pp. 1453-1460), IEEE.
S. Maldonado-Bascon, S. Lafuente-Arroyo, P. Gil-Jimenez, H. Gomez-Moreno, F. Lopez-Ferreras. Road-Sign Detection and Recognition Based on Support Vector Machines. Intelligent Transportation Systems, IEEE Transactions on, 8, 264-278, 2007.
Kingma, D.P.; Ba, J. Adam: A method for stochastic optimization. In Proceedings of the International Conference for Learning Representations (ICLR), SanDiego, CA, USA, 7–9 May 2015; pp. 1–15.
A. Paszke, S. Gross, F. Massa, A. Lerer, J. Bradbury, G. Chanan, T. Killeen,Z. Lin, N. Gimelshein, L. Antiga, A. Desmaison, A. Kopf, E. Yang, Z. DeVito,M. Raison, A. Tejani, S. Chilamkurthy, B. Steiner, L. Fang, C. Bai, S. Chin-tala: PyTorch: An Imperative Style, High-Performance Deep Learning Library. arXiv:1912.01703, 2019.
Article Statistics
Downloads
Metrics
Copyright License
Copyright (c) 2022 Babakulov Bekzod Mamatkulovich

This work is licensed under a Creative Commons Attribution 4.0 International License.
Individual articles are published Open Access under the Creative Commons Licence: CC-BY 4.0.