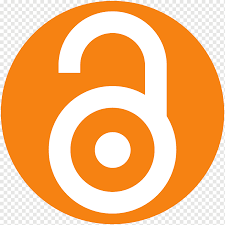
Machine Learning-Based Classification of Mental Health Status on Social Media: A Case Study of Kunduz, Afghanistan
Rohullah Adeeb , Department of Information Systems, Computer Science Faculty, Kunduz University, Afghanistan Hekmatullah Hekmat , Department of Information Systems, Computer Science Faculty, Kunduz University, Afghanistan Abdullah Zahirzada , Department of Information Systems, Computer Science Faculty, Kunduz University, AfghanistanAbstract
The proliferation of user-generated content results from social media's rapid development. An interdisciplinary field called computational cyber-psychology uses machine learning techniques to investigate fundamental psychological tendencies. Our study uses social media usage patterns to infer users' mental health status. The emergence of social media platforms in Afghanistan has profoundly affected the country's young people. This study aims to implement a predictive model using three machine-learning algorithms. The three selected algorithms are Naïve Bayes, Random Forest, and K-Nearest Neighbor (K-NN). The dataset contained in this study was collected through a questionnaire from students at Kunduz public and private universities in 2024. Data preprocessing is done before implementing the predictive models. The dataset is prepared carefully to ensure well-balanced samples in each category. The study reveals that Random Forest is the best classifier, with an accuracy of 77%. The study directly benefits the other researchers and policy or decision-makers in Afghanistan.
Keywords
Random Forest, Social Media, Mental Health
References
Hao, B., Li, L., Li, A., & Zhu, T. (2013). Predicting mental health status on social media: a preliminary study on microblog. In Cross-Cultural Design. Cultural Differences in Everyday Life: 5th International Conference, CCD 2013, Held as Part of HCI International 2013, Las Vegas, NV, USA, July 21-26, 2013, Proceedings, Part II 5 (pp. 101-110). Springer Berlin Heidelberg.
C. E. Montenegro, P. F. Geng, and R. P. Oliver, “Social media and mental health: A perspective on youth and online behavior,” Journal of Social Computing, vol. 12, no. 4, pp. 67-81, 2021.
Zahirzda, A., Chanmas, G., & Chan, J. H. (2023). A data mining model for predicting diarrhea in Afghan children. Authorea Preprints.
K. S. Ray, J. T. Hogan, and A. N. Kumar, “Comparative study of classifiers for mental health prediction on social media,” in Proc. IEEE Int. Conf. Machine Learning Applications (ICMLA), New York, USA, 2020, pp. 554-560.
Zahirzada, A., & Lavangnananda, K. (2021, January). Implementing predictive model for low birth weight in Afghanistan. In 2021 13th International Conference on Knowledge and Smart Technology (KST) (pp. 67-72). IEEE.
Zahirzada, A., Zaheer, N., & Shahpoor, M. A. (2023). Machine Learning Algorithms to Predict Anemia in Children Under the Age of Five Years in Afghanistan: A Case of Kunduz Province. Journal of Survey in Fisheries Sciences, 10(4S), 752-762.
Biau, G., & Scornet, E. (2016). A random forest guided tour. Test, 25, 197-227.
Altabrawee, H., Ali, O. A. J., & Ajmi, S. Q. (2019). Predicting students’ performance using machine learning techniques. JOURNAL OF UNIVERSITY OF BABYLON for pure and applied sciences, 27(1), 194-205.
Br, T. Der. (2022). The F-Measure Paradox The F-Measure Paradox. February.
Frank, E., Hall, M., Trigg, L., Holmes, G., & Witten, I. H. (2004). Data mining in bioinformatics using Weka. Bioinformatics, 20(15), 2479–2481.
Article Statistics
Downloads
Metrics
Copyright License
Copyright (c) 2025 Rohullah Adeeb, Hekmatullah Hekmat, Abdullah Zahirzada

This work is licensed under a Creative Commons Attribution 4.0 International License.
Individual articles are published Open Access under the Creative Commons Licence: CC-BY 4.0.